Check out our latest publication in Communications Engineering (Nature Portfolio)
The article is entitled « Machine learning unveils surface refractive index dynamics in comb-like plasmonic optical fiber biosensors »
This work was conducted by Hadrien Fasseaux, Médéric Loyez and Christophe Caucheteur and shows how Machine Learning can improve the implementation of TFBGs as practical biosensors
https://www.nature.com/articles/s44172-024-00181-9
The precise measurement of surface refractive index changes is crucial in biosensing, providing insights into bioreceptors–analytes interactions. However, correlating intricate spectral features, with these refractive index variations remains a persistent challenge, particularly in optical fiber gratings-based Surface Plasmon Resonance sensing. Here, we introduce a machine learning-based approach to address this ongoing issue. We integrate a regression model with gold-coated tilted fiber Bragg grating sensors. This enhances signal stability and precision, enabling a correlation between spectral shifts and refractive index changes. Our approach eliminates the need for individual sensor calibration, thereby bolstering the effectiveness and efficiency of the sensing layer. We demonstrate the model’s versatility by showcasing its efficacy across two data acquisition systems with different resolutions, allowing for comparative analysis and robustness enhancement. Its application in a biosensing experiment for insulin functionalization and detection, demonstrates how this breakthrough approach marks an advancement in real-time refractive index monitoring.
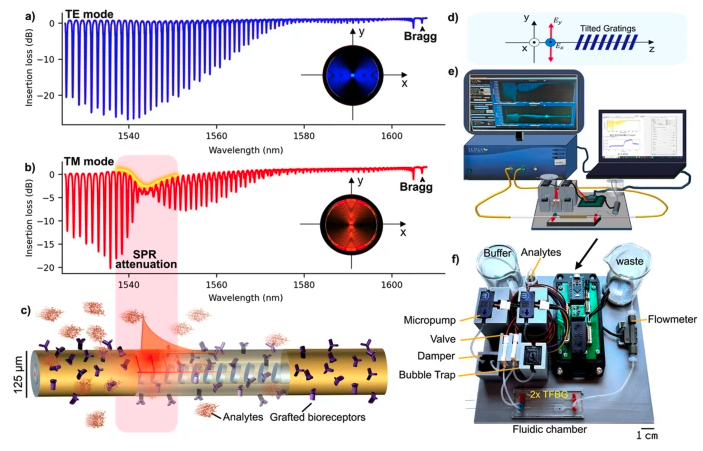